Our purpose
We believe that Scientific AI is the key to solving humanity’s grand challenges.
We know that the world’s scientific data, trapped in >10M silos, and in proprietary and unstructured formats, cannot effectively be exploited by AI.
We are catalyzing the Scientific AI revolution by designing and industrializing scientific AI-native data across the value chain.
We bring this AI-native data to life in a rapidly growing suite of next-generation lab data management solutions, scientific use cases, and AI-based outcomes.
We partner closely with our customers to drive transformational outcomes that cannot be achieved otherwise.
We have built a sui generis scientific data stack and company to accomplish all of this.
The hope and hype of Scientific AI
Scientific data, found in such vital industries as life sciences, chemicals, agriculture, materials, and energy, is among the world’s largest and fastest growing datasets and is upstream to the transformational breakthroughs required to solve humanity’s grand challenges.
The inexorable increase in computational power, democratization of cloud computing, and emergence of more powerful neural networks has led to much hope and hype in the realm of scientific discovery.
That said, in order for Scientific AI to become a commercial reality, large-scale, liquid, well-engineered, and compliant scientific datasets will need to be designed, assembled, and managed.
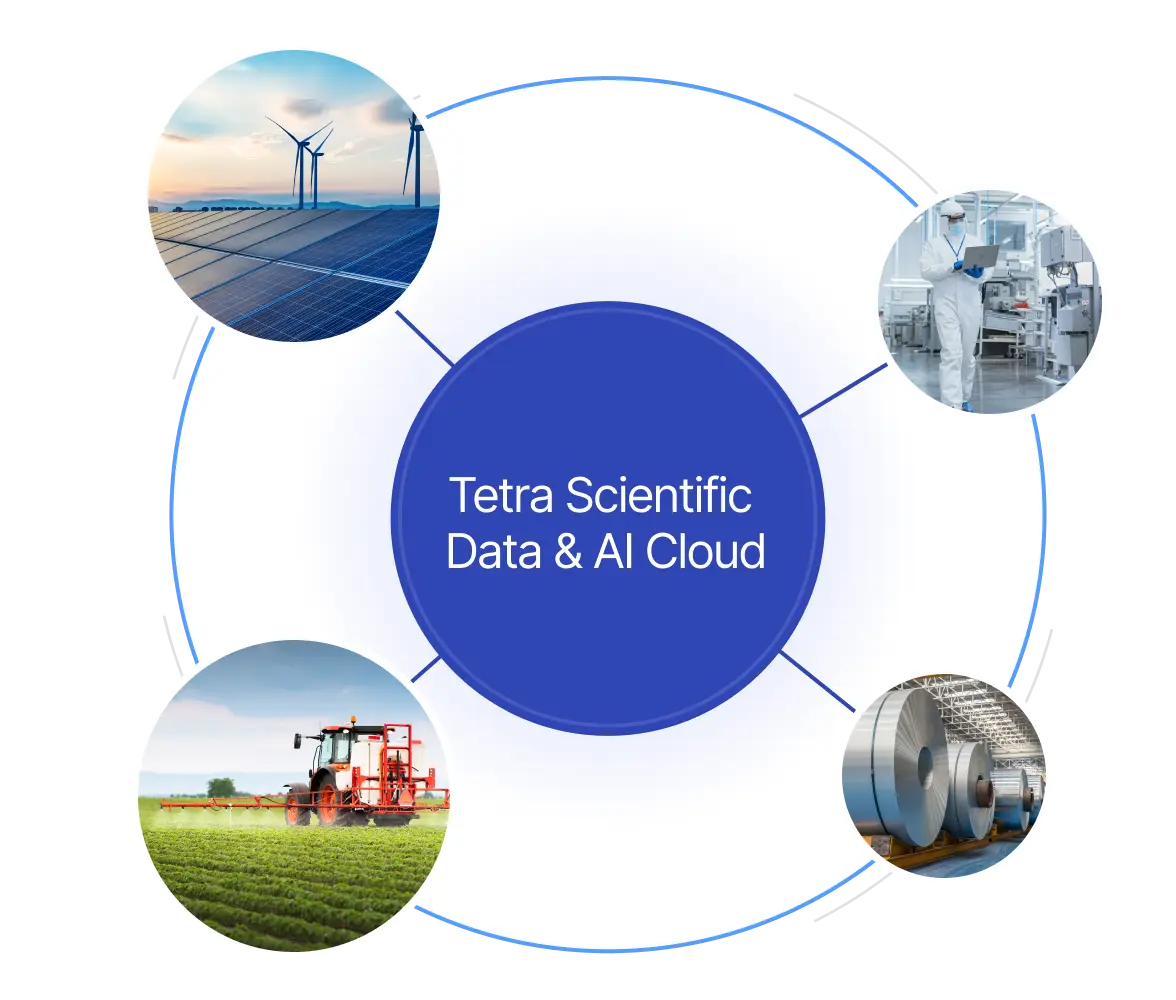
The scientific data silo quagmire
Today, the world’s scientific data is trapped in >10M silos, yielding subscale, proprietary, and/or unstructured data with no practical utility for predictive analytics, let alone AI. Four structural obstacles conspire to propagate these silos and inhibit data liquidity, data engineering, and AI utility.

Complex data and scientific use cases
Extraordinarily complex data and related scientific use cases and workflows, which require deep scientific expertise to understand and harness.
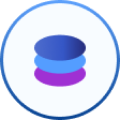
Fragmented scientific ecosystem
A highly fragmented scientific ecosystem of >500 vendors across instruments, electronic lab notebooks, informatics apps, robotics, IoT sensors, et al., and the attendant ingress, egress, and proprietary data complexity thereof.

Globally distributed value chain
A globally distributed value chain comprising tens of thousands of scientific customers and thousands of their contract research organizations (CROs) and contract development and manufacturing organizations (CDMOs), which materially exacerbates the scientific data silo and data model integrity dilemmas.

DIY approach to scientific data
A 20th century DIY project-based approach to scientific data within R&D labs and manufacturing/QC environments, which is typically disconnected from the actual scientific use cases, further impairing data utility.
TetraScience: Sui Generis
Our founders recognized that a sui generis approach — both technical and commercial — was required to enable the design and industrialization of scientific AI-native data.
To enable the assembly of large-scale and liquid scientific data sets, and to engineer sophisticated scientific data models comprising taxonomies and ontologies that are actionable by AI in furtherance of real-world scientific use cases, TetraScience has taken one-of-a-kind, full-stack approach.
Our approach
Science informs all we do
Each layer of the Tetra Scientific Data and AI Cloud is explicitly and optimally designed for scientific data.
Expertise in all aspects of scientific use cases and data, combined with parallel expertise in the modern data stack and AI, informs the design and development of TetraScience’s scientific data integration schemas, taxonomies, ontologies, partnerships, compliance standards, and AI models.
Embed Tetra Sciborgs
In addition to the scientific knowledge encoded in the TetraScience stack, our forward-deployed “Sciborgs” are embedded with customers and operate as one with their science and data science teams in pursuit of their scientific goals.
Tetra Sciborgs combine formal scientific expertise (e.g., Ph.D. in Molecular Biology; former biopharma bench scientist) with augmented data modeling training. They take the form of scientific data engineers and scientific business analysts.
Peer learnings and best practices
TetraScience combines its Sciborg engagements with a library of deployment-ready scientific use cases and supporting tools. This library includes access to customer peer learnings and benchmarking derived from its leadership position across the industry, in furtherance of accelerated and improved scientific discovery, development, and manufacturing.
Liberate and future proof your scientific data
TetraScience is the industry torchbearer for a vendor-agnostic data stack and data-only business model. Our only agenda is to liberate, harness, and future-proof the power of all our customers’ scientific data. All TetraScience resources—human, technical, and financial—are aligned against this objective.
Eliminate walled gardens and vendor lock-in
Conversely, scientific endpoint vendors (instruments, ELNs, informatics apps, robotics, IoT sensors, etc.) have vastly different competencies and agendas. They dabble in the modern data stack and issue press releases about AI but possess no core competencies or IP in either. They seek to use data-related offerings in an attempt to lock customers into proprietary walled gardens.
Enable data scale and liquidity for Scientific AI
Scientific endpoint vendors only have access to a small subset of the scientific data required to deliver on Scientific AI, whereas TetraScience, as the Switzerland of scientific data, secures access to the superset of our customer’s scientific data—a precondition for AI-native data.
Collaborate without data friction
Our customers’ replatformed and engineered scientific data—aka Tetra Data—is the atomic building block of Scientific AI and is designed to flow between and among customers and their CROs, CDMOs, and vendors, fueling unprecedented collaborative innovation.
Radically improve your data and AI outcomes
This unprecedented data liquidity fuels ever larger data scale, which leads to richer taxonomies, more robust ontologies, and higher fidelity AI-native data. Evermore liquid and improving AI-native data continuously improves Scientific AI models and outcomes.
Science informs all we do
Each layer of the Tetra Scientific Data and AI Cloud is explicitly and optimally designed for scientific data.
Expertise in all aspects of scientific use cases and data, combined with parallel expertise in the modern data stack and AI, informs the design and development of TetraScience’s scientific data integration schemas, taxonomies, ontologies, partnerships, compliance standards, and AI models.
Embed Tetra Sciborgs
In addition to the scientific knowledge encoded in the TetraScience stack, our forward-deployed “Sciborgs” are embedded with customers and operate as one with their science and data science teams in pursuit of their scientific goals.
Tetra Sciborgs combine formal scientific expertise (e.g., Ph.D. in Molecular Biology; former biopharma bench scientist) with augmented data modeling training. They take the form of scientific data engineers and scientific business analysts.
Peer learnings and best practices
TetraScience combines its Sciborg engagements with a library of deployment-ready scientific use cases and supporting tools. This library includes access to customer peer learnings and benchmarking derived from its leadership position across the industry, in furtherance of accelerated and improved scientific discovery, development, and manufacturing.
Liberate and future proof your scientific data
TetraScience is the industry torchbearer for a vendor-agnostic data stack and data-only business model. Our only agenda is to liberate, harness, and future-proof the power of all our customers’ scientific data. All TetraScience resources—human, technical, and financial—are aligned against this objective.
Eliminate walled gardens and vendor lock-in
Conversely, scientific endpoint vendors (instruments, ELNs, informatics apps, robotics, IoT sensors, etc.) have vastly different competencies and agendas. They dabble in the modern data stack and issue press releases about AI but possess no core competencies or IP in either. They seek to use data-related offerings in an attempt to lock customers into proprietary walled gardens.
Enable data scale and liquidity for Scientific AI
Scientific endpoint vendors only have access to a small subset of the scientific data required to deliver on Scientific AI, whereas TetraScience, as the Switzerland of scientific data, secures access to the superset of our customer’s scientific data—a precondition for AI-native data.
Collaborate without data friction
Our customers’ replatformed and engineered scientific data—aka Tetra Data—is the atomic building block of Scientific AI and is designed to flow between and among customers and their CROs, CDMOs, and vendors, fueling unprecedented collaborative innovation.
Radically improve your data and AI outcomes
This unprecedented data liquidity fuels ever larger data scale, which leads to richer taxonomies, more robust ontologies, and higher fidelity AI-native data. Evermore liquid and improving AI-native data continuously improves Scientific AI models and outcomes.