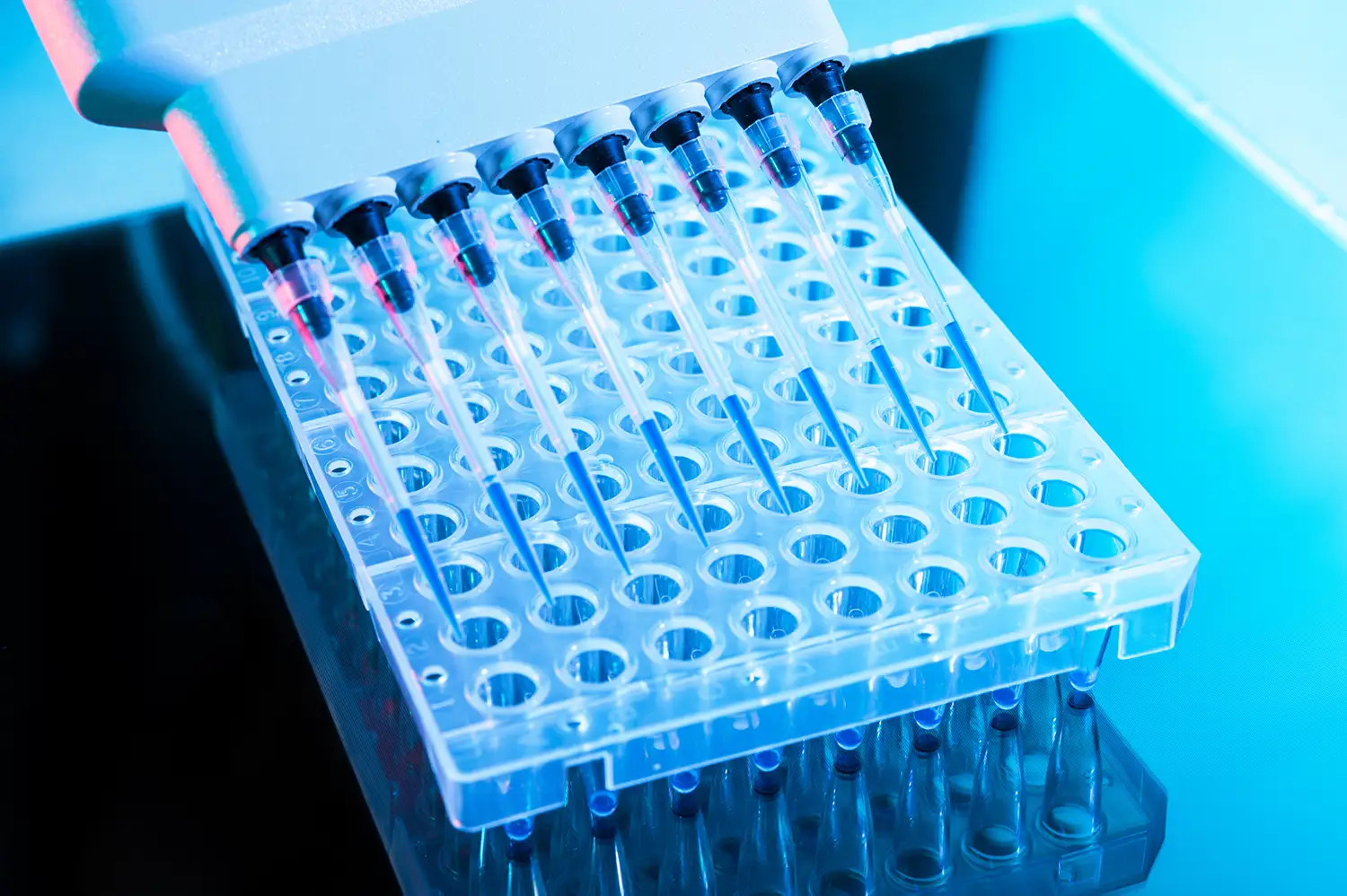
Accelerate and improve biologics characterization
Discover how to identify and monitor CQAs faster, better
Faster
process development
More accurately
defined and correlated CQAs and CPPs
Earlier
identification of deviations
The Rise of Sciborgs: Transforming How Science Gets Done
Introducing our new series: Sciborg™ Sessions. See how Sciborgs are fundamentally changing how science gets done.
Tune in live on LinkedIn:
February 27th | 11am ET