Tetra Benchling Connector, Round-trip Lab Data Automation and Contextualization of Instrument Data using Metadata from Benchling

Automating manual processes is one of the key improvements required in the biopharma industry to improve efficiency and throughput while reducing data collection errors when building complete scientific datasets without human intervention.
The power of harnessing scientific data lies within pairing laboratory instrument data from analytical systems with contextual metadata recorded in electronic lab notebooks (ELNs). Contextualization is the process of using experimental metadata to assign context and establish findability by answering the following questions: what is the data, who will use it, and how will it be used? Historically, the context describing a test was isolated in an ELN and required manual processes to identify, locate, and pair instrument raw data with experimental metadata.
The Tetra Benchling Connector, and other Tetra ELN connectors, bring that context into the Tetra Data Platform (TDP) and simultaneously drive round-trip lab automation of scientific workflows to provide a foundation for analytics and AI.
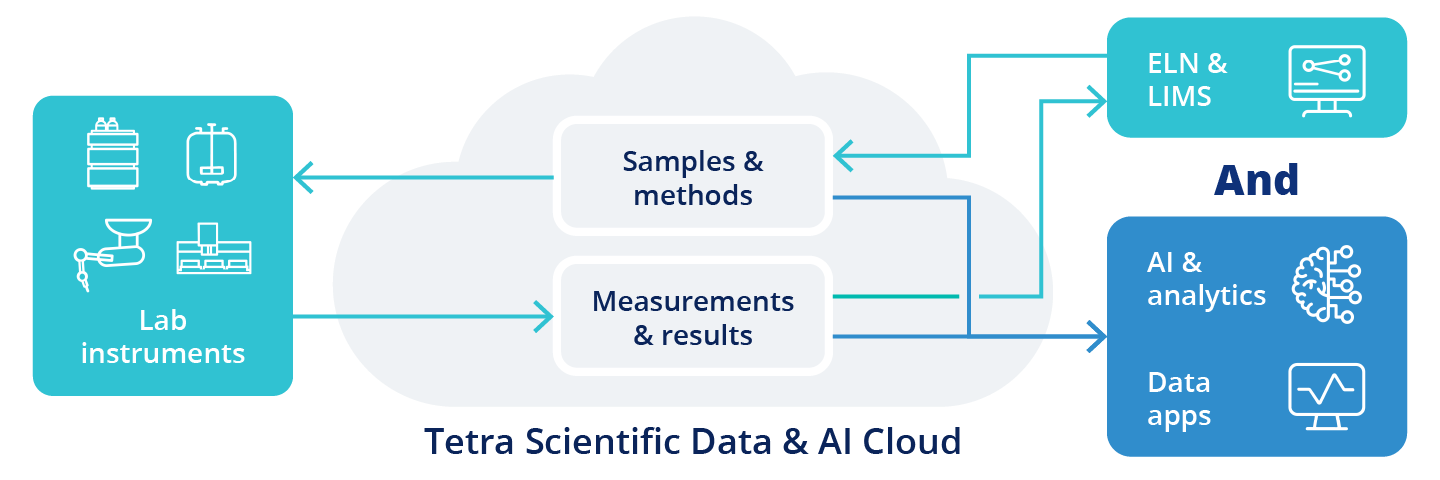
The Cost of Manual Processes
Scientific data makes many stops on the journey from experimental ideation to reported results. Scientists invest great effort into routinely moving data between systems during this journey using manual processes or point-to-point integrations. There are countless instances where manual processes are driving development of next generation therapeutics even in the world’s most innovative biopharma organizations. One industry-leading customer reported that 500 of their scientists collectively spend 5,000 hours a week entering chromatography data into ELN and chromatography data systems (CDS).
A recent study appearing in Drug Discovery Today determined that the current R&D efficiency to discover a new drug costs $6.16 billion. Biopharma is faced with the challenge of evolving their digital technology stack to lower drug discovery costs while meeting patient needs. One opportunity is onboarding solutions that enable full round-trip lab automation that eliminates manual data entry across biopharma systems. Round-trip lab automation is a seamless process where an experiment created in an ELN triggers a workflow that automatically sends test orders to instruments and reports assay results back to ELN. A scientist is enabled to invest their time, energy, and resources into high-value, innovative work instead of manual and routine data entry tasks.
TetraScience’s new Tetra Benchling Connector, paired with the TDP and Tetra instrument Agents, enables round-trip lab data automation for biopharma. Additionally, these tools help TDP contextualize instrument data using metadata siloed in Benchling Notebooks.
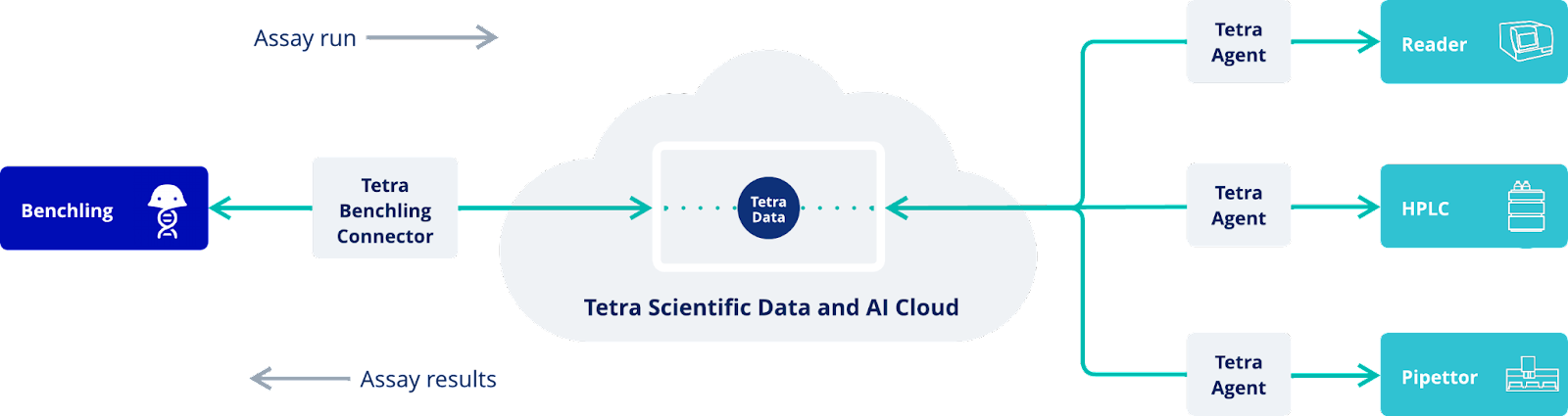
A Round-trip Lab Data Automation Journey for Chromatography Data
Chromatography serves as a pivotal technique in biopharma for the purification, characterization, and quality control of therapeutics. Typically, these instruments are managed by a CDS, like Thermo Fisher Scientific’s Chromeleon. A CDS connects multiple instruments across laboratories and provides a controlled environment for chromatography data analysis.
A major challenge that Biopharma scientists and IT staff face is creating a seamless flow of data from ELN to CDS and back again. Ultimately, scientists will rely on manual processes that transcribe scientific metadata from an ELN into a CDS, and they are forced to manually report chromatography results into ELN. These workflows result in data loss where the bare minimum results are captured and the remaining raw instrument data is siloed. The fact that most CDS data is available as a proprietary binary format further exacerbates these challenges.
Bidirectional data workflows using the Tetra Benchling Connector and Tetra Chromeleon Agent solve these challenges and bring round-trip lab automation into laboratory workflows. The following video will showcase these tools in action.
Benchling Notebook to ThermoFisher Chromeleon
The workflow begins when an operator initializes a Benchling assay run schema to start an experiment. An assay run schema defines the metadata tracked throughout an experiment’s execution and captures information like sample ID, experiment ID, and device ID. Next, the operator registers entities for injections and creates the Chromeleon sequence to select injections for the test. When the sequence is generated the Tetra Benchling Connector is activated and migrates the sequence to TDP. Notably, the arrival of the sequence file activates a series of pipelines that contextualize the file with scientific metadata from the assay run schema and convert it into an intermediate data schema (IDS). TDP leverages the new labels to surface information for easy searchability. Additionally, the pipelines send the sequence order to Chromeleon CDS. When the sequence arrives in Chromeleon, the scientist executes the injections and performs their regular peak integration analysis and result sign-off to complete the test.
ThermoFisher Chromeleon to Benchling Notebook
Result sign-off triggers the reverse process to send peak data into Benchling. A Tetra raw-to-IDS pipeline is activated when the results from Chromeleon arrive in TDP. Next, the IDS is transfigured into a processed result file that conforms to the Benchling result schema. Result schemas formally define what measurement data will be displayed in a Benchling Notebook. Notably, each pipeline creates a new file that is fully contextualized with appropriate scientific metadata to increase result searchability. Finally, a pipeline sends the processed results to the Benchling Notebook and the experiment is ready for formal review.
The combination of the Tetra Benchling Connector and Tetra Chromeleon Agents enables a fully automated lab data workflow where the operator’s job is now simplified to loading an instrument.
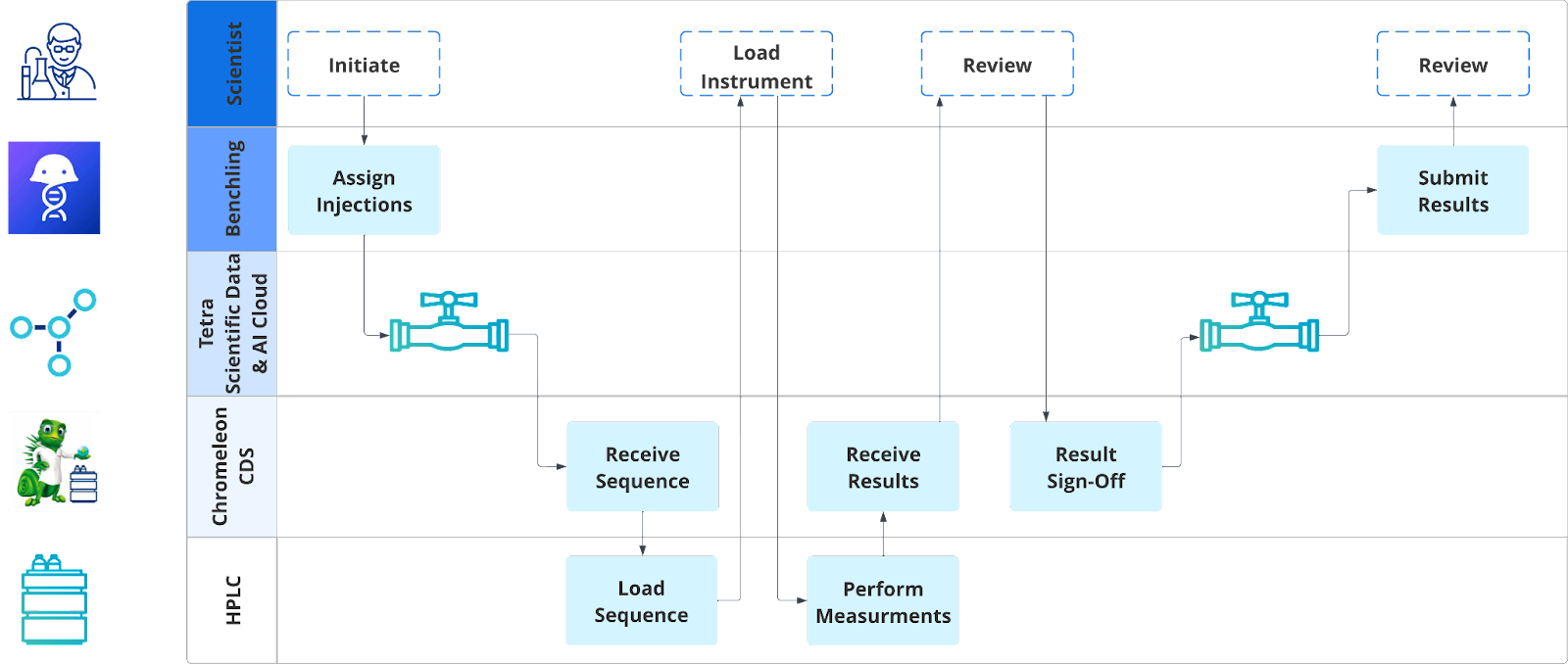
Contextualized Instrument Data
The immediate value of contextualizing raw instrument data is increased file searchability and connecting information across workflows. A standard multi-tenant deployment of the Tetra Scientific Data and AI Cloud will contain multiple organizations. Each organization will leverage numerous Agents and Connectors to capture data and various pipelines to harmonize and transform data. Scientific metadata can be extracted from Benchling’s assay run schema into an IDS and then contextualization pipelines are executed to label files. Notably, these contextualization pipelines can be deployed in a customized manner that is fine-tailored to meet individual customer user requirements. This is an important caveat because each customer has a unique implementation of their ELN and CDS environments.

Labels extracted from the assay run schema are then used to search for data by various conditions. In this use case, the Benchling entity label for injection ID was added to the Chromeleon generated files. Performing a label based search for “INJ009” will return all files (e.g., Benchling assay run IDS, Chromeleon IDS, Peaks.csv, and Benchling result schema) associated with that particular label.
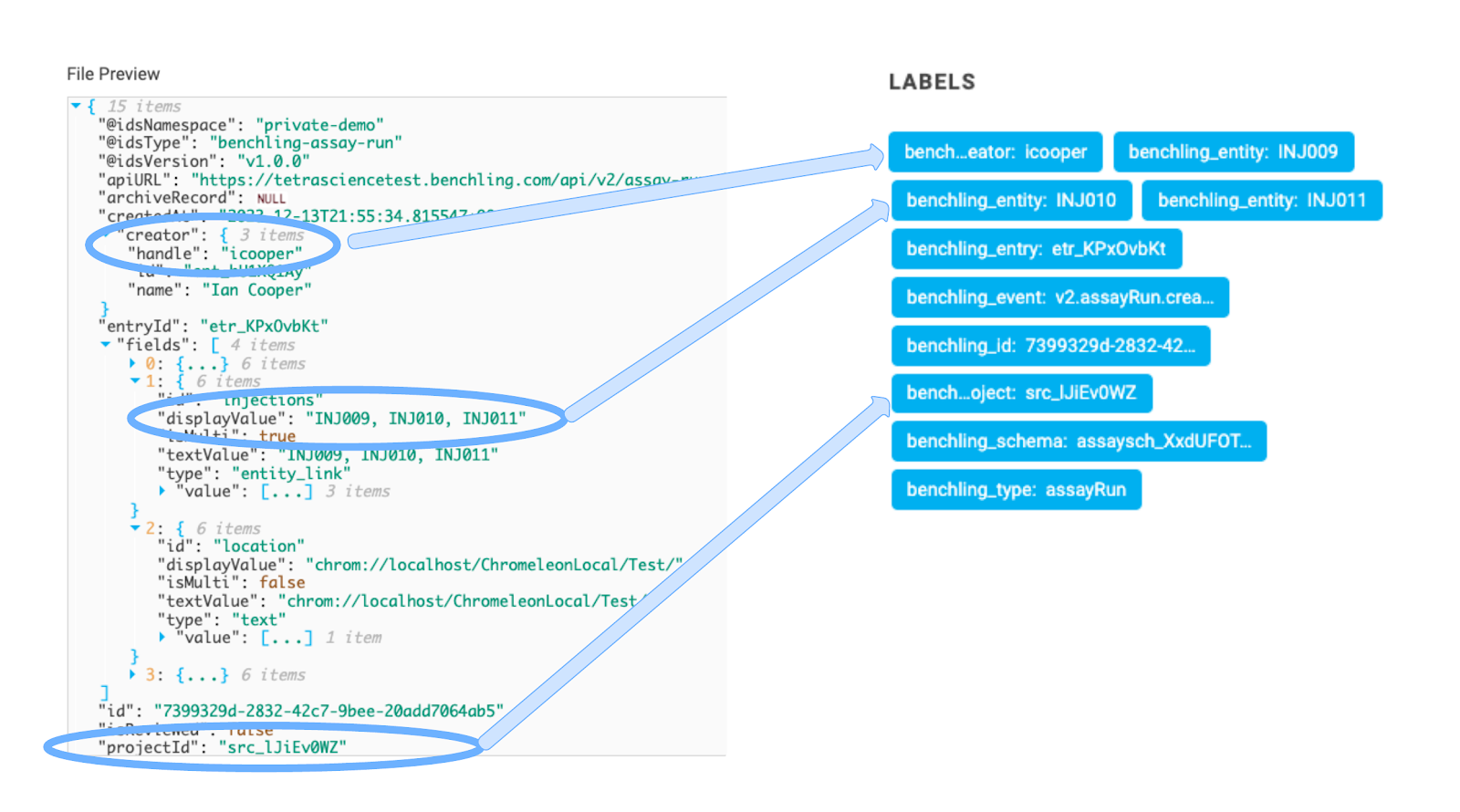
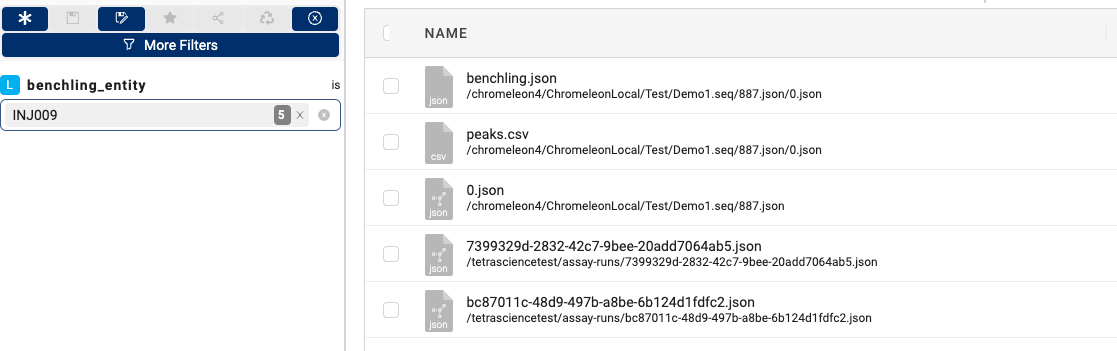
The use case above highlights a simple value realization of contextualized instrument data. Bidirectional integration expands ELN notebook capabilities to include hard-to-surface data points like instrument ID or column ID. Instrument and column identifiers are recorded in CDS systems for each injection performed but manually transcribing that information from CDS to ELN is a tedious and error-prone task. However, the Tetra Chromeleon IDS readily captures and surfaces that information through IDS harmonization and file labeling. Bidirectional integrations ensure that information can be captured in an ELN notebook too! A list of select contextual data use cases are provided in Table 1. Future articles will dive deeper into contextualized instrument data analytics and AI applications.
Table 1. Contextual Data Use Cases
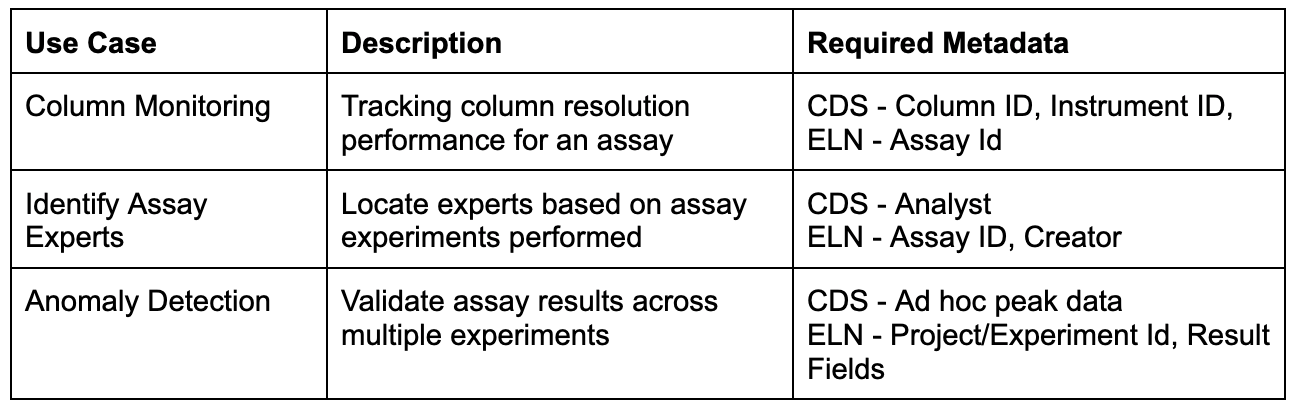
Lab Data Automation and Foundation for Analytics/AI
Round-trip lab automation provides immediate, data replatforming (layer 1) value to biopharma organizations and the unquestionable business value of reallocating scientists from data entry tasks towards strategic work. Contextualization helps advance biopharma into layer 2, data engineering, where data science teams now have the appropriate metadata to search and query scientific data across their organization to begin designing custom datasets. Round-trip lab automation and contextualization improve overall operating efficiency and elevate biopharma into data analytics (layer 3) and AI (layer 4) layers of the data journey pyramid.
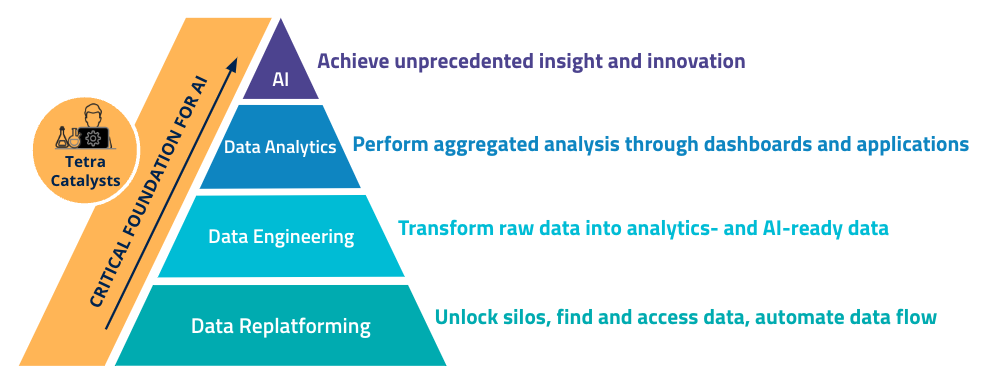
Data science teams are already developing AI applications to automate chromatography data analysis tasks like peak detection and peak integration (Anal. Chem. 2020, J. Chromatogr. A. 2022, Bioinformatics 2022). The challenge these teams face is fine-tuning peak detection models using their own custom chromatography data sets. A custom data set for model development often requires a scientist to navigate through a CDS to locate the appropriate data and export it using a copy-and-paste procedure. Additionally, the scientist is required to navigate their ELN to ensure the appropriate metadata is included in the training set. This type of approach often forces data science teams to settle with the initial data set created for training and they are prevented from building an optimal data set for training purposes. If the data science team desires to continuously improve their model using a human-in-the-loop mechanism then they are forced to retroactively perform this process on a recurring basis.
One top-25 biopharma customer has developed their own chromatography peak detection model with human-in-the-loop functionality for a reduced phase, ion phase (RP-IP) assay. The human-in-the-loop component is triggered when model detected peaks fail to pass quality thresholds and scientists are required to perform manual peak detection and integration in CDS. A new ELN entry is created to collect the manually integrated peak data from failed analyses and the data is added to a training set to further refine model performance. Notably, the scientists are also required to export custom CDS files to capture the chromatogram raw and peak data for model training purposes.
The Tetra Scientific Data and AI Cloud is able to drastically improve this manual process by providing round-trip lab automation and contextualization. An experiment failure automatically activates the human-in-the-loop process, described above, using TDP to automatically create re-annotation worklists, capture and contextualize scientist integrated chromatogram data. A combination of file versioning and appropriate ELN contextualization data can be used to differentiate model integrations from scientist integrations, and a series of pipelines can be created that automatically add scientist integration data to the appropriate training data sets. This type of capability transforms a model re-training strategy from a critical mass approach to an active learning approach. A critical mass strategy is when a modeling team waits until they have collected enough data to justify re-training efforts. Active learning is a modeling technique that introduces new training data into a model in real-time to continuously improve the model’s predictive capability and domain. Roundtrip lab automation is estimated to provide the customer approximately $5 million savings for one assay by eliminating manual data transfers between Benchling and Chromeleon. But the value proposition extends well beyond the reduction of operation expense, once you capitalize on the Genius of "And".
The Tetra Scientific Data and AI Cloud is helping the pharmaceutical industry accelerate scientific discovery and development with harmonized, contextualized, and AI-ready scientific data in the cloud. Reach out to TetraScience scientific data architects and scientific business analysts to learn more about how your organization can leverage the Tetra Scientific Data and AI Cloud and the newly released bidirectional Tetra Benchling Connector to support Notebook-to-Notebook integrations.