We’re excited to feature Melax Tech and Dr. Frank Manion, Vice President for Innovations, as part of our Spotlight Series on emerging companies and technologies and highlight their use of Natural Language Processing (NLP) to solve real-world problems from unstructured data sets and documents.
.png)
Please tell us about Melax Tech.
Melax Tech is a Houston-based company specializing in applying biomedical Natural Language Processing (NLP) to solve real-world problems involving data and information extraction from unstructured text-based documents. We are world leaders in the development of NLP technology for applications in biopharma and health care. Our NLP workbench products allow users to rapidly build custom NLP applications for their needs and support state-of-the-art NLP methods employing Deep Learning and Machine Learning approaches. We support both named entity recognition (NER) and relation extraction (RE), as well as entity normalization with major biomedical terminology systems. In addition to our workbench products, we have pre-built, end-to-end applications supporting target discovery, clinical trials, and economic feasibility in the pharmaceutical industry based on large-scale scientific literature mining and knowledge integration.
We are world leaders in the development of NLP technology for applications in biopharma and health care.
For the biomedical research community, our technology is used to extract information from peer-reviewed articles, patents, and clinical trials and to relate this data to knowledge bases in the biomedical domain. For pharmaceutical R&D teams, we have built tools to automate literature reviews by providing “live” systematic literature reviews and meta-analysis tools. We have tools supporting cohort discovery, and complex patient cohort matching to clinical trial protocols.
For pharmaceutical R&D teams, we have built tools to automate literature reviews by providing “live” systematic literature reviews and meta-analysis tools.
For healthcare and population health applications, we support large-scale information extraction of documents typically associated with Electronic Health Records, such as physician notes, laboratory results, radiology reports, hospital discharge summaries, etc. The unstructured data from these documents is known to constitute about 80 percent of the available information in medical records and is thus missed by systems focusing solely on structured data fields. The data we extract can be used for all sorts of purposes, such as enhancing clinical care by detecting early indications of disease, or by matching patients to appropriate clinical trials on the basis of complex phenotype and genotype criteria, prior treatments, and failed therapies.
An infographic of our current suite of products is shown below.
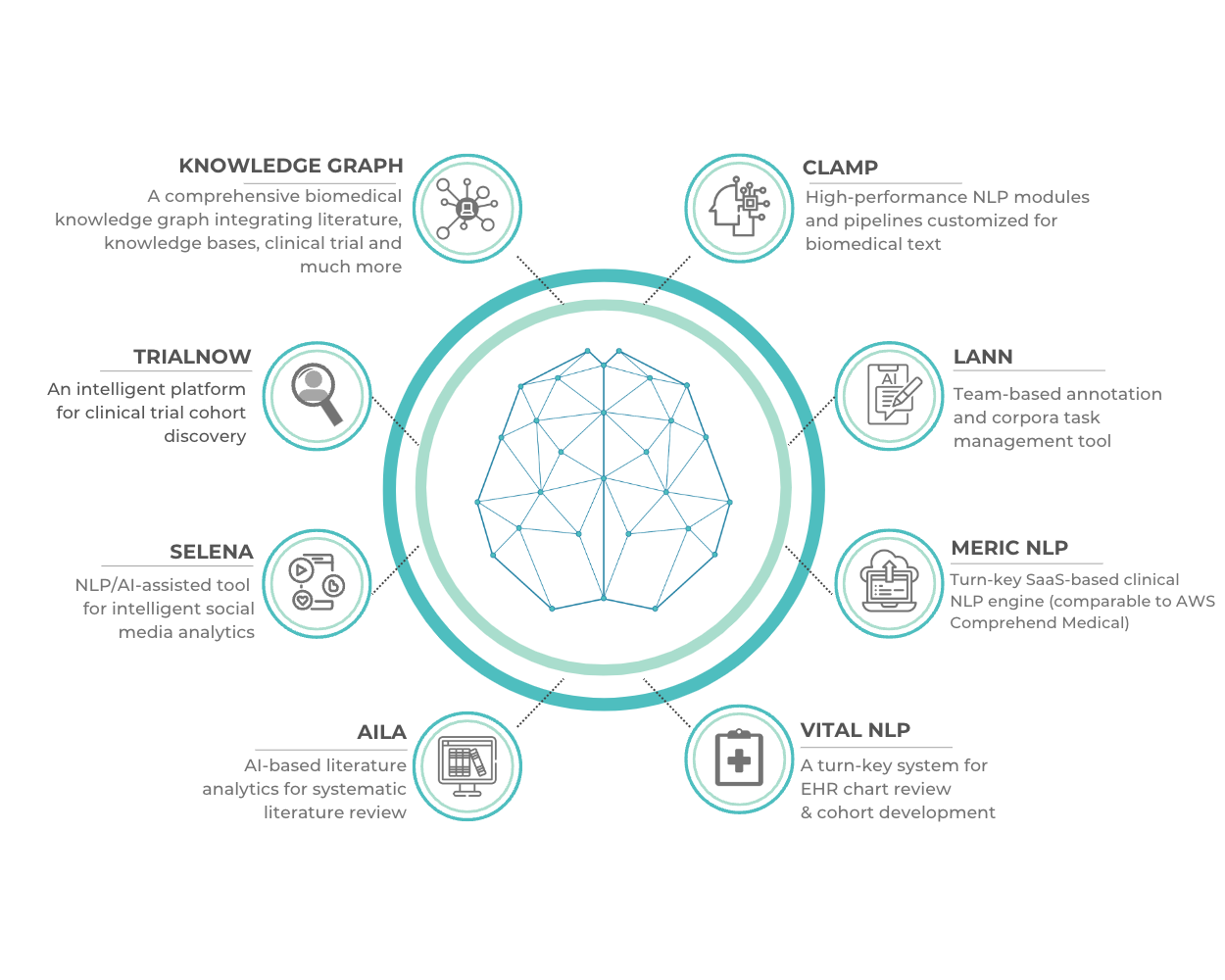
Please tell us a little bit about your background and that of your team.
I have a late-career Ph.D. in biomedical informatics from the University of Texas Health Science Center at Houston School of Biomedical Informatics, where my research focused on the use of ontologies and formal semantics to address problems in biorepository research and the regulatory environment surrounding it. Before joining Melax Tech, I served as the Chief Informatics Officer at the Rogel Cancer Center at the University of Michigan and as the CTO for Fox Chase Cancer Center in Philadelphia, where I was heavily involved in large-scale human genome initiatives and the development of research cyberinfrastructure.
The Melax Tech team has eight members with Ph.D. 's in clinical NLP and formal semantics, and many of our colleagues have master's degrees in computer science.
The Melax Tech team has eight members with Ph.D. 's in clinical NLP and formal semantics, and many of our colleagues have master's degrees in computer science. Our executive team has strong experience in business, management, and scaling SaaS companies. The company evolved out of the laboratory of Dr. Hua Xu at the School of Biomedical Informatics at the University of Texas in Houston, and the core members of our technical team have all been in the field of NLP for at least 13 years. Our early work developed named entity recognition for clinical texts, and we have since participated in many NLP challenges for extraction of clinical texts, where our algorithms often take the top spots, as shown in the figure below.
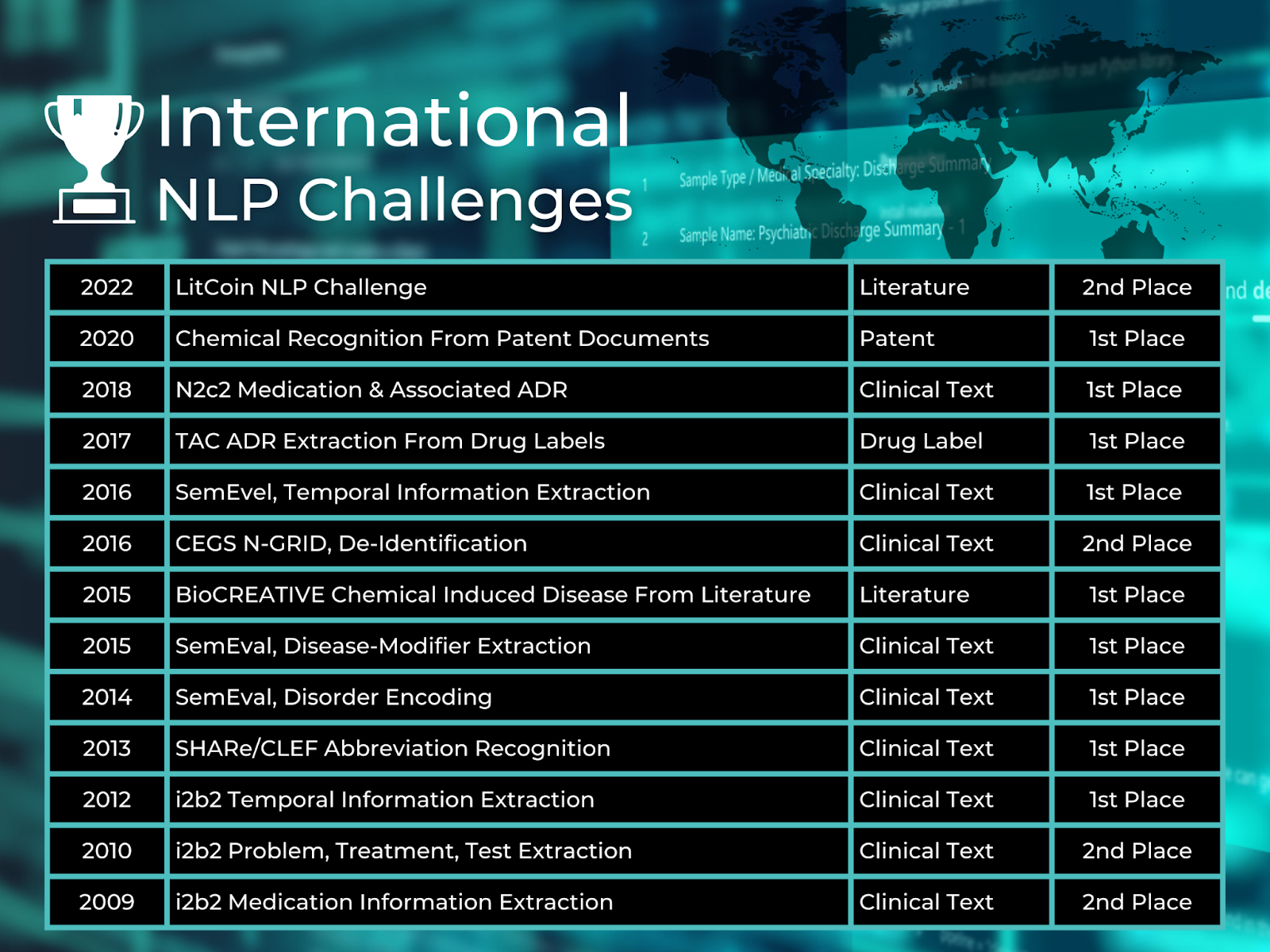
Melax has participated in numerous clinical NLP challenges for the extraction of clinical texts where the company’s algorithms were top-ranked for various tasks
Can you explain Natural Language Processing (NLP) and how it’s solving real-world problems in biopharma research?
Natural Language Processing is a branch of Artificial Intelligence and Computer Science that applies algorithms and AI-driven approaches to derive data and information from text. The text is processed into “named entities,” such as the name of a laboratory test, a lab reagent, a person’s name, etc., and these are labeled with a suitable tag. Relations between named entities are also recognized, such as a DNA sequence encoding for a specific protein. The results of the NLP process are typically then further used with deep learning or machine learning approaches to address specific real-world use cases.
We are currently partnering with major biopharmaceutical companies to use our biomedical knowledge graphs. Knowledge graphs were originally invented by Google to enhance their search engine with information gathered from a variety of sources. They encode structured information of entities and relationships within a network. The Melax knowledge graph contains over 700,000 unique entities (e.g., disease, gene, chemical) and 43 million relations from literature and other important biomedical resources.
The Melax knowledge graph contains over 700,000 unique entities (e.g., disease, gene, chemical) and 43 million relations from literature and other important biomedical resources.
Our biomedical knowledge graph will help customers get answers to frequently asked questions such as: What is the biological mechanism (relationships with gene, protein, chemical) for a particular disease and where does the formation come from (e.g., which paper, which sentence)? Are there “treatment” relationships between one known drug with diseases that are out of original indication?
How are biopharma companies using systematic literature reviews to accelerate research?
Systematic literature reviews (SLRs) are a major methodological tool in many areas of the health sciences. They are essential in helping biopharmaceutical companies understand the current knowledge about a topic and identify research and development directions. In the field of health economics and outcomes research (HEOR), researchers routinely conduct SLRs to understand the research landscape, synthesize evidence about unmet medical needs, compare the economic value of various treatment options, and prepare the design and execution of future real-world evidence studies.
Systematic literature reviews (SLRs) are a major methodological tool in many areas of the health sciences.
Conducting an SLR 1) involves synthesizing high-quality evidence from biomedical literature in a transparent and reproducible manner, 2) seeks to include all available evidence on a given research question, and 3) provides some assessment regarding the quality of the evidence. Currently, an SLR is often conducted manually, which is resource-consuming from both the labor and financial perspectives. A recent study found that each SLR costs approximately $141,195 to conduct, and the ten largest pharmaceutical companies publish about 119 SLRs a year for a total cost of roughly $16 million per year per company. Further, SLRs are known to go out of date quickly – up to 15% are out of date within one year.1 Since SLRs are critical to developing treatment guidelines in the clinic and setting research priorities in the biopharmaceutical industry, developing robust automated systems to keep them up-to-date is a priority.
As biomedical literature grows at an unprecedented rate, advanced artificial intelligence solutions are an excellent approach to expedite SLR efforts. Consequently, a concept known as a Living Systematic Review is emerging where AI-based systems assist with the literature review by screening abstracts and full text nearly continuously, resulting in high-quality, up-to-date online summaries of health research that are updated when important new research becomes available. Our AI-based Literature Analysis (AILA) tool is a product that provides SLRs targeted to certain areas of Health Economics and Outcomes Research (HEOR). AILA employs a human-in-the-loop architecture and can produce Living Systematic Reviews.
As biomedical literature grows at an unprecedented rate, advanced artificial intelligence solutions are an excellent approach to expedite SLR efforts.
Our technology also can be applied to clinical trial protocol documents to understand which criteria are needed to enroll patients into specific clinical trials. The data from the patients and the clinical trial protocols can be used by a hospital or pharmaceutical company to find patients who may be eligible for a particular clinical trial. NLP has been used for a wide range of applications, including disease surveillance, pharmacovigilance, and clinical decision support.
The easiest way to understand NLP technology and how it can save you time, money, and headaches is to try it. Sit in on a brief demo with our customer support team by requesting a demo.
___________________________
1 Michelson, M. & Reuter, K. The significant cost of systematic reviews and meta-analyses: A call for greater involvement of machine learning to assess the promise of clinical trials. Contemporary Clinical Trials Communications 16, 100443 (2019)