Smart quality control (QC) aims to enhance QC processes in the biopharmaceutical industry through the integration of automation, advanced analytics, and artificial intelligence (AI). According to a McKinsey report, average-performing QC labs could achieve up to a 200% improvement in productivity by adopting these technologies. Furthermore, using AI and analytics to augment decision intelligence could reduce lead times by up to 70%.
Yet, despite this compelling vision, biopharma has been slow to reap these benefits.

Challenges in Implementing Smart QC
A common belief is that the daunting costs associated with the smart QC evolution are the main obstacles, particularly the high maintenance required to make fragmented QC data ready for analytics and AI. However, the root of this challenge often lies deeper: organizations lack a product-led approach to building AI systems. They frequently focus on technology for its own sake rather than integrating it into a coherent strategy that addresses specific scientific and operational needs.
For instance, some biopharma companies have implemented generative AI (GenAI) to analyze QC data but found that these solutions give inconsistent responses or fail to provide actionable insights. This often happens when the AI solutions are developed in isolation from the end users—QC and QA personnel—without fully understanding the complexities of the QC processes or the regulatory requirements involved. Without a product-led approach that begins with the users' needs and scientific context, these AI initiatives can become costly projects that deliver little value, leading to frustration and wasted resources.
A Science-Grounded, Product-Led Approach
At TetraScience, we believe the key to smart QC is building science-centric, data-driven products that unlock the power of GenAI while delivering deterministic, reliable answers.
The foundation for this approach is a large-scale, high-fidelity dataset to model biopharma QC. Our product design uses three categories of data:
- Evidence: Deterministic values obtained from scientific instruments and verified calculations, such as phenotypic metrics (e.g., titer), genotypic data, or genomic traits. These quantifiable and qualifiable data points form the objective basis for quality assessments. Sometimes, this information is recorded as handwritten observations in a structured form like a lab worksheet or batch record.
- Knowledge: Decision-making processes such as investigation workflows and standard operating procedures (SOPs) within the QA and QC domains. It also covers less structured information such as technical reports, investigation reports, or tribal knowledge from experienced QC professionals.
- Regulatory Requirements: Guidelines and mandates from regulatory bodies such as the U.S. Food and Drug Administration (FDA) and the European Medicines Agency (EMA). They set the compliance standards for QC processes to ensure product safety and efficacy.

Building on this framework, we develop ontologies, taxonomies, and schemas that guide our product development. Ontologies define the concepts and relationships within our data domain, taxonomies classify and organize these concepts into hierarchical structures, and schemas specify how the data is structured and formatted. By integrating these elements, we ensure a structured and accurate representation of the data and processes to support AI models effectively.
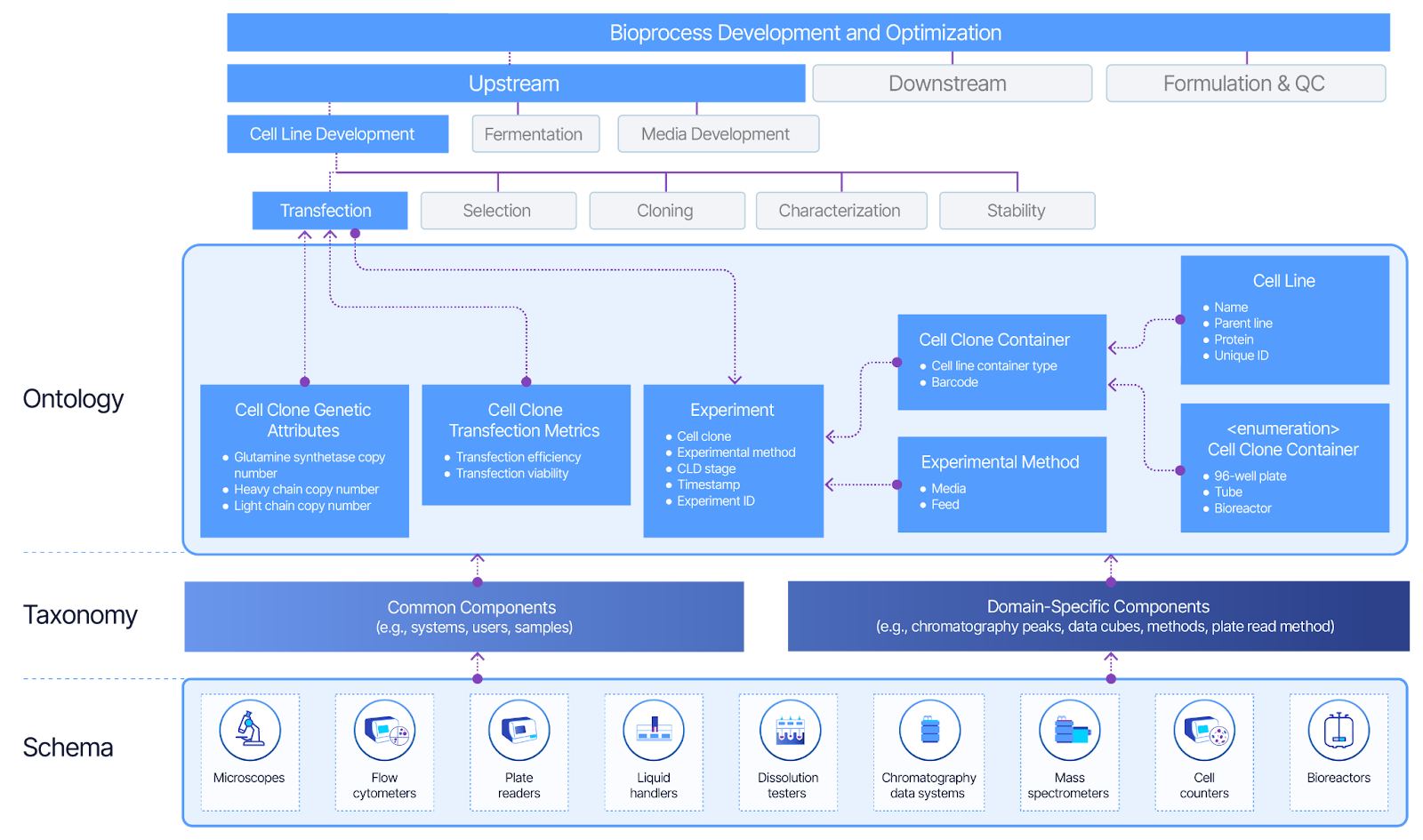
Use Case: GenAI with QC Reports
To illustrate the value of a product-led approach, we’ll examine the challenges of quality control in gene therapies and then present a pilot use case that uses GenAI to address these issues.
QC Challenges in Gene Therapy
Gene therapy represents a revolutionary approach in precision medicine, offering potential cures for a range of genetic diseases. By directly modifying or replacing faulty genes within a patient's cells, gene therapies have the potential to treat conditions previously considered incurable. The unique characteristics of gene therapies, however, present significant challenges in QA, QC, and batch release.
One of the primary challenges stems from the diversity and complexity of gene therapy modalities. These therapies can use viral vectors (e.g., adeno-associated virus [AAV]), non-viral delivery systems (e.g., lipid nanoparticles), and genome-editing tools (e.g., CRISPR/Cas9). Each modality has distinct manufacturing processes, stability profiles, and safety considerations, making standardization difficult.
Batch release testing is more demanding for gene therapies than for traditional drugs due to the higher number of (smaller) batches and the variable nature of the biological materials involved. This added complexity can lead to delays during QC, which is particularly problematic given the limited stability of gene therapies and the life-threatening nature of many patients’ conditions.
The evolving guidelines from regulatory bodies like the FDA and EMA introduce further uncertainty. As these agencies update their regulations to keep pace with rapid advancements in gene therapy technologies, organizations must continuously adapt their QA and QC processes to remain compliant.
These complexities necessitate a more dynamic and intelligent approach to QC. Recognizing this, Andelyn Biosciences, a gene therapy contract development and manufacturing organization (CDMO), partnered with TetraScience to implement smart QC solutions within its operations.
Our Product-First Approach in Action
To tackle the challenges of gene therapy QC, we plan to embed GenAI models—grounded in evidence and knowledge—into Andelyn’s processes. These models will enable QC scientists and leaders to analyze complex datasets and prepare reports with much greater speed and accuracy than ever before. Here’s an overview of our approach:

- Ontology Development. We curated evidence and knowledge sources following the 5M QC framework (man, machine, method, material, measurement). We created detailed ontologies and schemas to model the relationships between different stages within the batch release workflow. This ontology, combined with standardized data formats, ensures consistency across enterprise search, extending beyond QC into areas like supply chain and sales.
- Data Integration. Aligning with Andelyn’s vision for universal data access and storage throughout the organization, we aim to automate the unification and harmonization of metadata definitions from diverse data sources, such as their lab instruments (e.g., droplet digital PCR), laboratory information management system (LIMS), quality management system (QMS), and enterprise resource planning (ERP) system. We also will incorporate FDA guidelines for investigational new drug (IND) filings relevant to gene therapy.
- Designing Agentic Systems. We designed AI agents to autonomously perform specific tasks using tools like LangChain. These agents transform unstructured batch release reports into structured evidence and knowledge, allowing the AI to parse complex documents and extract critical data points essential for QC processes.

- Fine-Tuning Models with Scientific Context. We fine-tuned a foundational large language model (LLM) model—Google’s Gemini Pro—guided by the ontology and QC context gleaned in the prior steps. This included developing high-quality optical character recognition (OCR), layout parsers, and custom entity extractors from unstructured QC reports, which are augmented with scientific evidence harmonized in the Tetra Scientific Data and AI Cloud™.

In our pilot, this product-first approach produced GenAI models with an average F1 score of 0.90, indicating high accuracy and reliability:
Next Steps: Enhancing Scientists’ Reasoning
We aim to create knowledge nodes that turn correlated data into causal inferences. This will enable GenAI to answer complex questions like: “What are the main root causes of out-of-spec deviations if I start this AAV5 batch release?”
This pilot focuses on a human-in-the-loop approach, using AI to assist rather than replace human expertise. By implementing this GenAI solution in QC processes, we expect to improve the handling of unstructured and multimodal data, leading to enhanced accuracy and efficiency in data extraction.
This data can feed into other AI and analytics models, resulting in increased throughput, reduced deviation closure time, and lower costs. More efficient, cost-effective, and responsive QC operations will enhance overall business performance and benefit patients in urgent need of these treatments.
Closing Remarks
By adopting a science-grounded, product-led approach to integrating GenAI into QC processes, we can overcome the challenges hindering smart QC adoption. Our pilot demonstrates that careful ontology development, data integration, and model fine-tuning can create AI systems that provide reliable, actionable insights and help accelerate time to patient.