In 2016, humanity entered the zettabyte era, which is a fancy way of saying that the micro-nuggets of information floating around the global data sphere had finally reached one trillion gigabytes. This massive number took us about two decades to reach, but yet more dramatic growth is still ahead. By 2025, in just three years, we will be at 175 zettabytes. That’s right—the data creation train is moving at breakneck speed, and it’s not slowing down any time soon, nor is the growing complexity of scientific data.
What does this data explosion mean for life sciences?
We must radically rethink how to harness and manage this colossal amount of complex scientific data effectively. And that process starts with accepting what we all know, but don’t want to admit. The existing solutions we’ve relied upon to date - brittle point-to-point integrations, data lakes and data clouds not built for scientific purposes, or expanded use of LIMS, ELN, or other legacy systems with proprietary “walled gardens” - can’t sustain us anymore.
There are some parallels in the area of scientific data with the history of smartphones and cell phones. The rise of Apple (smartphones) and the downfall of Nokia (flip-phones) wasn’t because Nokia failed to innovate; it’s that Nokia limited its innovation to incremental improvements within the confines of existing solutions. Had it sought to solve its customers’ biggest problems, Nokia’s story may have turned out differently.
Legacy Data Management Falls Short
Biopharma scientists need to unlock the full value of their data in ways that fuel therapeutic innovation, achieve faster time-to-market for safe and effective new treatments, and optimize quality with advanced analytics and AI/ML. But with the current systems in place, scientists have to jump through several frustrating hoops to make it happen. The three “Ghosts of Data Past” that haunt the users and gatekeepers of scientific data are:
- Data are scattered across a mix of on-premises environments (i.e., legacy systems that are relics from another time and can’t scale to today’s needs)
- Files are regularly shared on an ad hoc basis, which limits visibility across teams
- Data in these files are heterogeneous and often locked in vendor-proprietary formats
Consider a common scientific workflow:
- Scientists produce data and reports from biochemical assays
- Results pass through quality checks or enrichment
- If the data check out, they are further pushed to ELN or LIMS
- If an issue arises, stakeholders are notified to take action and rectify the data mistake with full traceability
- Data are also parsed to enable data visualization and data science
If you’re a researcher or data scientist, efforts to collect and prepare these data are often costing you 50% of your time or more. Beyond the fact that data pruning and transformation is exhausting, soul-numbing work, it’s also robbing you—and your organization—of the valuable time you could be using to connect insights, innovate, and bring new therapies to the market that will improve patients’ lives (which is probably why you got into the business in the first place).
So, let’s take a deeper look into how life science has allowed itself to be inundated by its data.
We’re still beholden to 20-year-old legacy solutions
Legacy solutions do a decent job of capturing and archiving data from scientific instruments (HPLCs, mass specs, flow cytometers, sequencers, etc.) and scientific applications (LIMS, ELN, or analysis software). They’re data warehouses with limited workflows around data management. In the early 2000s, these solutions were great! They were a huge step up from maintaining paper reports (remember the quaintness of filing cabinets?). But as data has exploded, these legacy solutions can’t keep up. As a result, they’ve created scientific data "black holes"—troves of data forgotten and unfindable later, locked away in silos, systems, and ad hoc file shares. They’re also in hundreds of different formats and schemas, many of which, as we noted, are vendor proprietary.
That leaves our researchers and data scientists with the messy and error-prone job of decoding and harmonizing the various data outputs they need to run advanced analytics or AI/ML for their study. Also keep in mind that labs are full of important data sources that are not in the form of files, like blood gas analyzers, chromatography data systems, and more. You need integrations that go beyond what legacy systems can achieve, but until you master those, manual data transformation is your only option.
Remember that 50% statistic? It’s starting to make sense, right? Let’s all agree right here and now that our researchers and data scientists deserve better than flip-phone-era data management. Okay, moving on…
Let’s talk about scale
Because many legacy solutions are on-premises or use limited cloud storage as data centers, scalability challenges abound. Remember we mentioned the zettabyte era earlier on? As scientific workflows and modalities become more complex, chemistry and assay outputs on the 100MB-scale are giving way to proteomes, genomes, images, and exome maps. No legacy solution on the market today has the processing capabilities or scalability required to handle the volume and complexity of this scientific data deluge.
As a result, legacy users have to divide and move data across a multitude of applications, systems, and collaborators. There’s no seamless data flow and no universal access across teams. There’s also no support for GxP, making regulatory audits time-consuming. Without a way to restore all historical data, efforts are duplicated and searchability is poor.
Finally, consider that on-prem legacy solutions typically require several modules, like databases, servers, and file storage, all of which need to be modified with every single upgrade. I’m getting tired just thinking about that endless cycle.
Thankfully, there’s a better way.
Introducing the Tetra Scientific Data Cloud
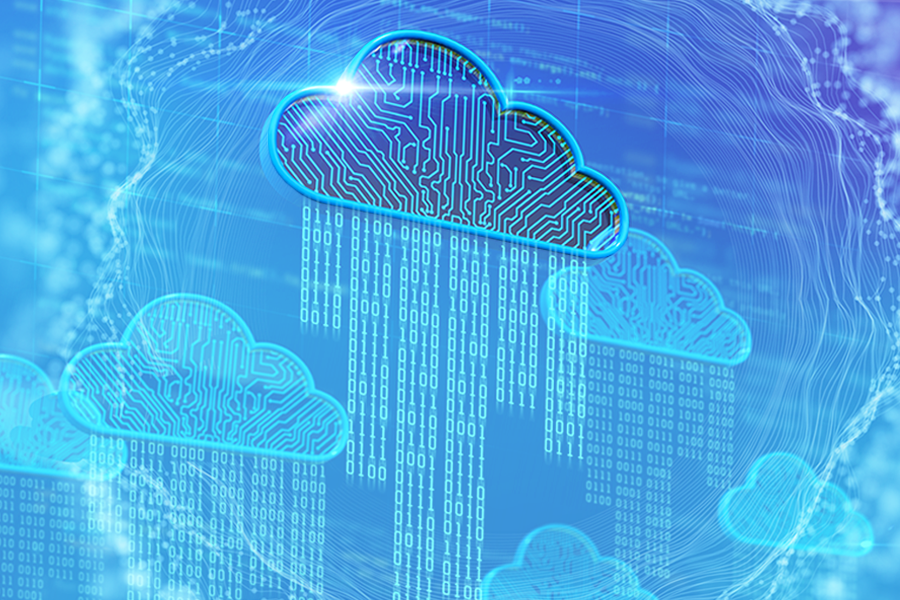
Now that we’ve talked through what’s been going wrong in data management, let’s talk about what we need to make it right.
Modern science requires data liquidity, meaning we need to make this whole concept of “data silos” a thing of the past, starting now. Scientific data are competitive currency, and legacy systems are like piggy banks already filled to the brim, leaving huge amounts of value uncaptured. That’s why you need cloud-native solutions built to scale elastically, where data can flow seamlessly across all your instruments and informatics apps, speeding your time to insights. FAIR (Findable, Accessible, Interoperable, and Reusable) data should be the new standard—but if we want to disrupt life sciences, that’s not enough. To truly supercharge the power of your data across R&D, manufacturing, and QA/QC, we need to go further.
We need to harmonize them in an open, vendor-agnostic format, freeing scientists from the arduous process of manually reconstructing datasets so they speak the same language. Data should also be enriched with metadata for scientific context, unlocking your ability to summon the data you need with a simple browser-like search. And, in a perfect world, all of your historical data should be simple to find and restore, taking the headache out of audits with a complete audit trail.
With the Tetra Scientific Data Cloud, we’ve brought this holy grail of scientific data management to life.
The Tetra Scientific Data Cloud distinguishes itself from traditional solutions by breaking down barriers previously constraining your laboratory ecosystem. It is:
- The only open, vendor-agnostic platform with a connected digital ecosystem of suppliers
- The only cloud-native platform that engineers compliant, harmonized, liquid, actionable data (we call Tetra Data) to support the entire scientific data journey
- The only platform purpose built for scientific data across R&D, manufacturing, and QA/QC with data fluency across diverse scientific domains
You gain increased team efficiency with simple-to-manage, productized integrations; better access and reliability with automatic data flow across ELNs and informatics applications; and greater innovation through actionable data prepared for advanced analytics, visualization, and AI/ML. The cloud-native infrastructure is also cost efficient since you won’t need to shell out money to maintain and upgrade out-of-date systems. The Tetra Scientific Data Cloud is the next-generation data solution, and it’s a game changer.
The Bottom Line
Right now, there’s a massive global movement across biopharma to replatform in the cloud, and the reason is simple: legacy solutions are simply not good enough anymore.
Once Apple debuted the iPhone and customers realized what they were missing, the Nokia flip phone went from somewhat passé to utterly archaic in what felt like a nanosecond. At its height in 2007, Nokia owned 50.9 percent of the global market share. By 2010, that number dropped to 27.6 percent, and in 2013 (with a market share of 3.1 percent), it sold its mobile device business entirely to Microsoft.
Don’t wait—unburden yourself from the “Three Ghosts of Data Past” and position your company for rapid innovation, faster time to market, and optimized quality across your teams now with the Tetra Scientific Data Cloud. You’ll thank us later!